Vehicle manufacturers and suppliers need sophisticated tool chains for the verification and validation of new driveline technologies and automated driving functions. These tool chains should allow the integration of tools from different vendors. Each individual tool in the chain needs access to a specific set of annotated data. This is particularly true when it comes to safety argumentation and homologation of vehicle functions.
In order to avoid the disadvantages of individual data silos for each verification and validation tool, experts suggest to establish ASAM ODS in the companies as a reliable and future-proof basis for implementing a comprehensive "Test Data Lake" approach. The major strength of ASAM ODS as compared to non-standardized data storage solutions is its highly adaptable data model (= base model) and a well thought-out HTTP-API. The data model provides a proven classification for test data and features for managing the meaning and storage location of bulk data. The HTTP-API makes the access to this data independent of certain IT architectures, technologies and vendor-specific tools. From the ASAM ODS point of view it doesn´t matter whether the organization´s data management approach involves one or more data sources at one or several locations. There are also no specific runtime environments and file storage platforms required by ASAM ODS. And, it doesn´t matter whether ASAM ODS is operated on-premises, on a public cloud or in the context of a hybrid approach. To put it in other words: The basic ideas and concepts of ASAM ODS can be used within a wide range of application areas and technical infrastructures, including big data and cloud ecosystems. This ensures that test data do not disappear forever in "big data graveyards" and can be used by many users and different tool chains in the long term.
But how can such an overall, ODS-based approach to test data management be implemented in a company? A suitable starting point is the use of the Eclipse open source platform openMDM 5.
First of all, openMDM 5 provides companies a pre-defined, but individually adaptable and extensible ODS-based data model (= application model), allowing the users to systematically manage all the information and data sets necessary for the verification, validation and homologation of vehicles, components and functions. The data to be managed may include for example organizational information toward a test, test case and test step descriptions, test parameters, test setups, sensor data in a variety of formats, logging and trace data, test results and reports, and many more.
Secondly, openMDM 5 offers a well-defined data access layer (= openMDM API) that encapsulates the technical details of ASAM ODS and thus enables the various applications within a tool chain to access the required test data objects in a targeted manner. In this way, tool suppliers can for example integrate their products into existing tool chains with manageable effort.
And finally, openMDM 5 comes along with a web-based user interface that user companies and tool providers can use and expand for the implementation of their own special use cases with regard to test data navigation, search, interpretation, visualization, selection and provision/exchange.
In addition to this, a large number of ASAM ODS and openMDM 5 compliant tools and solutions are available on the market, facilitating the implementation of an overall test data management solution. An example of this is the Peak Data Transfer Tool, which can be used to import different data types and formats into the data model of ASAM ODS/openMDM. During the import the data can be cleaned, harmonized or supplemented via plugins. A standardized "Calculation Processor" additionally allows the calculation of new values based on Python scripts. Another tool is the Peak Spark ODS Adapter. Test and data engineers use this tool to access ODS and associated bulk data (e.g. stored in a Hadoop system) in such a way that they can be easily used both in a variety of analysis scripts (e.g. based on Python, Matlab or Diadem ) as well as within "off-the-shelf" analytical frameworks (like PowerBI, Tableau and others).
Basically with this, companies have everything they need to be able to pursue a vendor and tool-independent approach for an overall test data management for verification, validation and homologation of vehicles. Ultimately, it is up to the management to recognize the benefits of such an approach for the own company and to promote its implementation based on concrete use cases.
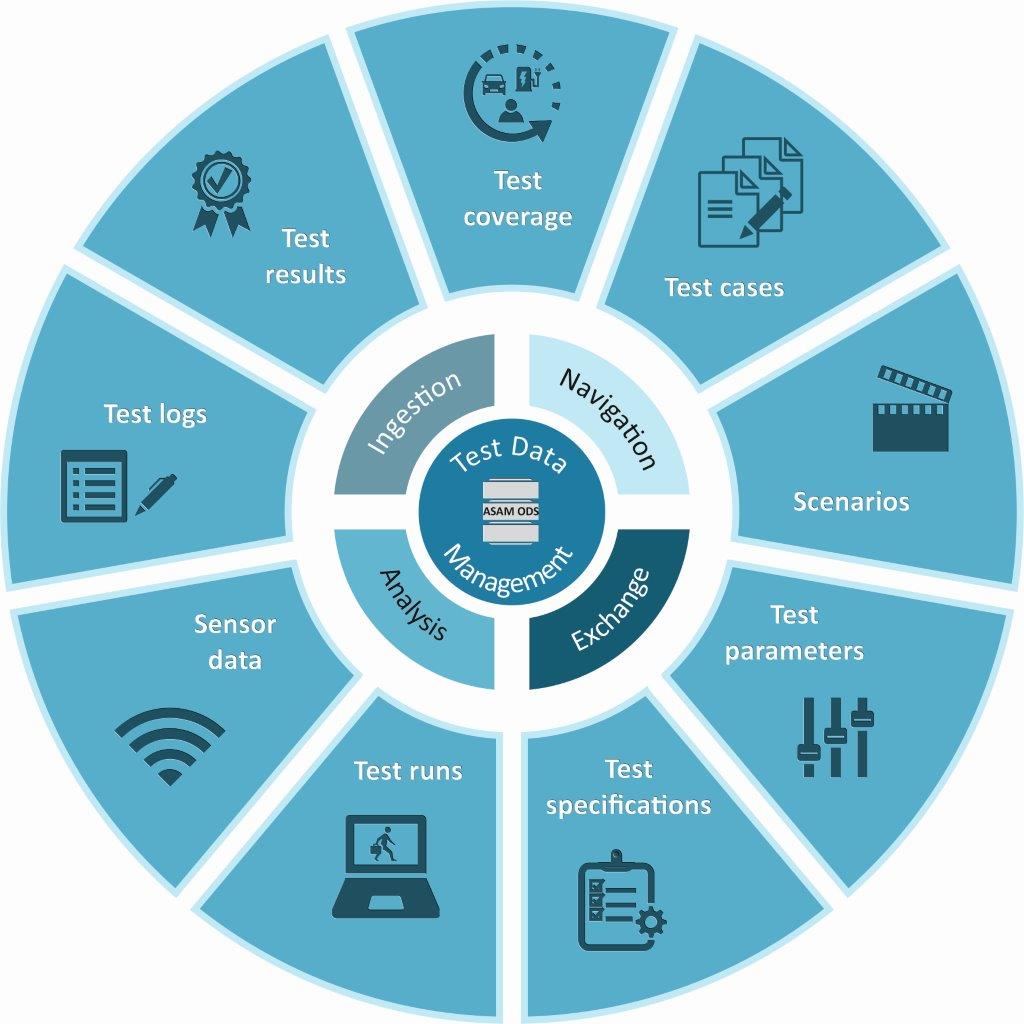